Abstract
Background
Mobile health (mHealth) apps can be used for cardiovascular disease (CVD) prevention. User-centered design, evidence-based content and user testing can be applied to ensure a high level of usability and adequate app access.Objective
To develop and evaluate an mHealth app (HerzFit) for CVD prevention.Methods
HerzFit�s development included a user-centered design approach and guideline-based content creation based on the identified requirements of the target group. Beta testing and a preliminary usability evaluation of the HerzFit prototype were performed. For evaluation, German versions of the System Usability Scale (SUS) and the mHealth App Usability Questionnaire (GER-MAUQ) as well as free text feedback were applied.Results
User-centered design thinking led to the definition of four personas. Based on their requirements, HerzFit enables users to individually assess, monitor, and optimize their cardiovascular risk profile. Users are also provided with a variety of evidence-based information on CVD and their risk factors. The user interface and system design followed the identified functional requirements. Beta-testers provided feedback on the structure and functionality and rated the usability of HerzFit�s prototype as slightly above average both in SUS and GER-MAUQ rating. Participants positively noted the variety of functions and information presented in HerzFit, while negative feedback mostly concerned wearable synchronization.Conclusions
The present study demonstrates the user-centered development of a guideline-based mHealth app for CVD prevention. Beta-testing and a preliminary usability study were used to further improve the HerzFit app until its official release.Free full text

User-centered development of an mHealth app for cardiovascular prevention
Abstract
Background
Mobile health (mHealth) apps can be used for cardiovascular disease (CVD) prevention. User-centered design, evidence-based content and user testing can be applied to ensure a high level of usability and adequate app access.
Objective
To develop and evaluate an mHealth app (HerzFit) for CVD prevention.
Methods
HerzFit´s development included a user-centered design approach and guideline-based content creation based on the identified requirements of the target group. Beta testing and a preliminary usability evaluation of the HerzFit prototype were performed. For evaluation, German versions of the System Usability Scale (SUS) and the mHealth App Usability Questionnaire (GER-MAUQ) as well as free text feedback were applied.
Results
User-centered design thinking led to the definition of four personas. Based on their requirements, HerzFit enables users to individually assess, monitor, and optimize their cardiovascular risk profile. Users are also provided with a variety of evidence-based information on CVD and their risk factors. The user interface and system design followed the identified functional requirements. Beta-testers provided feedback on the structure and functionality and rated the usability of HerzFit´s prototype as slightly above average both in SUS and GER-MAUQ rating. Participants positively noted the variety of functions and information presented in HerzFit, while negative feedback mostly concerned wearable synchronization.
Conclusions
The present study demonstrates the user-centered development of a guideline-based mHealth app for CVD prevention. Beta-testing and a preliminary usability study were used to further improve the HerzFit app until its official release.
Introduction
The most effective way to prevent cardiovascular diseases (CVDs) is to promote a healthy lifestyle and to encourage the control of modifiable risk factors. 1 However, in reality, the prevalence of unhealthy lifestyle is high, and many people lack adequate control of risk factors, even when they are at high cardiovascular risk. 2 Modern concepts are needed to address unhealthy lifestyle habits and poorly controlled risk factors. Mobile health (mHealth) apps might be helpful in this regard by broadening the reach of healthcare providers and engaging individuals in health management. 3 In particular, the high prevalence of smartphones in groups with lower socioeconomic status promises to overcome traditional barriers to CVD preventive measures. 4
In recent years, there has been a steady increase in the number of apps addressing CVD risk factors such as diabetes mellitus (DM), hypertension (HTN), and lifestyle habits such as physical inactivity, smoking, unhealthy diet, and stress.5–10 Even though randomized trials reported improvements in lifestyle and risk factor control, the effects of mHealth apps on disease prevention and outcomes remain controversial.11–15 One of the reasons for the inconsistency in reported results might be the varying quality of mHealth apps. A recent consensus statement on the prevention of noncommunicable diseases pointed out the importance of human-centered design processes to develop effective and innovative interventions.16,17 Yet, human or user-centered design thinking approaches are still underutilized in the mHealth sector. 18 Another reason for the varying results might be the lack of evidence-based content in mHealth apps. 14 Two recent studies reviewing apps in the fields of dermatology and microbiology found that only a minority of the apps had medical professional involvement.19,20 Finally, a possible lack of usability evaluation could also explain the varying results of mHealth apps. Indeed, the number of studies reporting usability results has not increased to the same extent as the number of available mHealth apps. 21
The aim of the present study was the user-centered development and evaluation of an evidence-based mHealth app (HerzFit) for CVD prevention. HerzFit�s content was built on the portfolio of the scientific advisory board of the Deutsche Herzstiftung (German Heart Foundation), an organization with currently more than 100,000 members and close links to the German and European Cardiac Societies as well as the Deutsche Hochdruckliga (German Hypertension League).
Methods
The development study of the HerzFit app was separated into two phases: the conception and implementation phase and a subsequent beta testing and usability study of our prototype. Both phases involved close collaboration with representatives of the cooperating patient foundations as well as the potential target group of HerzFit.
Conception and implementation
The conception and implementation phase of HerzFit started in September 2020 and was finalized in January 2022. This phase included the requirements elicitation, the design of the user interface (UI) as well as the system design and the technical development of the app and its surrounding system.
Requirements elicitation and UI design
To meet the requirements of the potential target group, the design thinking method was applied. Design thinking describes a user-centered design approach that seeks effective interventions to address the needs identified.16,22,23 As proposed by the Hasso-Plattner Institute of Design at Stanford (d.school), design thinking comprises five steps: “empathize” (understand the users), “define” (identify goals and scope), “ideate” (create ideas), “prototype” (mock-up potential solutions), and “test” (gather feedback from test users).17,24 For this development phase, an interdisciplinary team was formed consisting of physicians from the German Heart Center Munich, medical informaticians and sports scientists from the Technical University of Munich, members of our cooperating patient foundations and app designers. In the first step of design thinking, members of the German Heart Foundation were interviewed in order to better understand our potential target group. In the second step, our interdisciplinary team defined four personas and their requirements. In the third step, we used these personas to create ideas for the goals, structure, functionality, and UI of the HerzFit app. In the fourth and fifth steps, these ideas including low-fidelity paper-based UI mockups were discussed and further elaborated with a group of potential users who were invited to join our interdisciplinary design thinking process. The group of test users consisted of three individuals without CVD, two individuals with heart disease, one individual with cerebrovascular disease, and one patient with congenital heart disease. Based on their feedback, the UI was iteratively developed, refined, reviewed, and improved by the interdisciplinary HerzFit team until the end of the conception and implementation phase of HerzFit.
System design and technical development
The system design started after the requirements elicitation and UI design. It included the identification of important technical requirements and architectural components based on the functional and nonfunctional requirements elicited, as well as the conception of an overall system architecture. Next, the technical stack was selected, including the programming languages, frameworks, and databases used in HerzFit and the surrounding systems. The implementation of HerzFit´s components was started in January 2021. To enable parallel development for both Android and iOS users, HerzFit was developed as a cross-platform app using the React Native library (Version 0.72) and written in TypeScript. For deployment and continuous delivery, the Expo/Expo Go system was used. The implementation followed an agile, iterative approach: every week, a new release of the most up-to-date version of HerzFit was made available to the interdisciplinary team. The technical development was finalized in January 2022.
Beta testing and usability study
In the second phase of HerzFit´s development, a combined beta testing and usability study of the HerzFit prototype was conducted between February and April 2022. For this purpose, 57 test users recruited through the channels of the German Heart Foundation and the German Hypertension League were invited via email to use the HerzFit prototype. No specific inclusion or exclusion criteria were defined, as the test users were recruited via the patient foundations and were therefore considered to be representative of HerzFit´s potential target group. Study participants only had to be in possession of a smartphone and be able to download the HerzFit prototype. Study participation was voluntary and written informed consent was provided for each participant.
In the first step, study participants were asked to use the HerzFit prototype for one week. In a second step, they received an invitation to an online questionnaire distributed via LimeSurvey. The questionnaire consisted of 47 questions in four categories: (1) general information about the user themselves, their relationship to CVD and their prior smartphone/wearable usage behavior, (2+
3) two usability questionnaires, the German versions of the System Usability Scale (SUS) and the mHealth App Usability Questionnaire (GER-MAUQ), and (4) more detailed questions on positive and negative aspects of the HerzFit app as part of the beta testing.25–27 The SUS—a simple 10-item test that allows to evaluate the usability of any system—has been shown to be the most frequently used questionnaire in mHealth applications.
21
The original version of the GER-MAUQ, which has been developed to specifically evaluate the usability of mHealth applications, consists of 18 questions divided into three subscales (Ease of Use, Interface and Satisfaction, and Usefulness) and has been shown to be a reliable and valid tool for usability evaluation.
26
The open questions of part 4 were chosen to get more detailed insights into parts and features of the app that users specifically liked or disliked, as well as problems that prevented them from using specific features of the app. This kind of feedback would not have been available from the results of the usability questionnaires of part 2
+
3.
21
Statistical analysis
Of the 57 test users, 50 submitted the questionnaire and were thus included in the usability evaluation. The responses for the usability questionnaires ranged from 1 (strongly disagree) to 5 (strongly agree) for the SUS and from 1 (strongly disagree) to 7 (agree) for the GER-MAUQ. A descriptive analysis of the demographic data and overall outcome was performed. For this purpose, the MAUQ overall score, its subscale scores, and the means and standard deviations were calculated with a higher GER-MAUQ score indicating greater usability.26,27 Given the 7-point Likert scale, a GER-MAUQ score of 4 was considered an average result. For analysis of the SUS, the overall score was determined and converted into a value between 0 and 100 using the standard conversion method. A SUS score above 68 was considered above average. 28 Statistical analysis was performed with the SPSS version 29.0.0.0.
Data availability
The data that support the findings of this study are available from the corresponding author upon reasonable request.
Results
Conception and implementation
Personas and functional requirements
To better understand the needs of HerzFit´s target group, four personas were defined: two patients with or at risk for CVD, one family member of a heart patient, and one physician (Figure 1). The needs of the personas were subsequently translated into four main functional requirements for HerzFit: (1) assessing individual cardiovascular risk, (2) monitoring health parameters, (3) improving lifestyle, and (4) providing information about CVD and their risk factors.
UI design
According to the four main functional requirements, the HerzFit app is divided into four core modules: two modules for assessing cardiovascular risk and monitoring health parameters (“Measure”), one module for lifestyle modification (“Improve”), and one module for information (“Learn”). As general UI elements, a combination of a darker and a lighter orange and a dark blue was chosen as the color scheme. A variety of icons were integrated in order to limit the amount of text and enable easier recognition of UI elements.
On the first app open, the app begins with an interactive onboarding process. After being introduced to the app, its core components and the data security concept, users are asked for their sex, age, height and weight. In the final step of the onboarding process, users can connect HerzFit with the operating system-specific health data storage (e.g. HealthKit on iOS) to allow automated data transfer into the app (Figure 2). After completing the onboarding process, users enter the main interface with its taskbar displaying HerzFit´s core modules “Measure,” “Improve,” and “Learn.” An additional profile area allows users to manage their profile settings and upload documents and pictures (e.g. recent medical reports) (Figure 3).
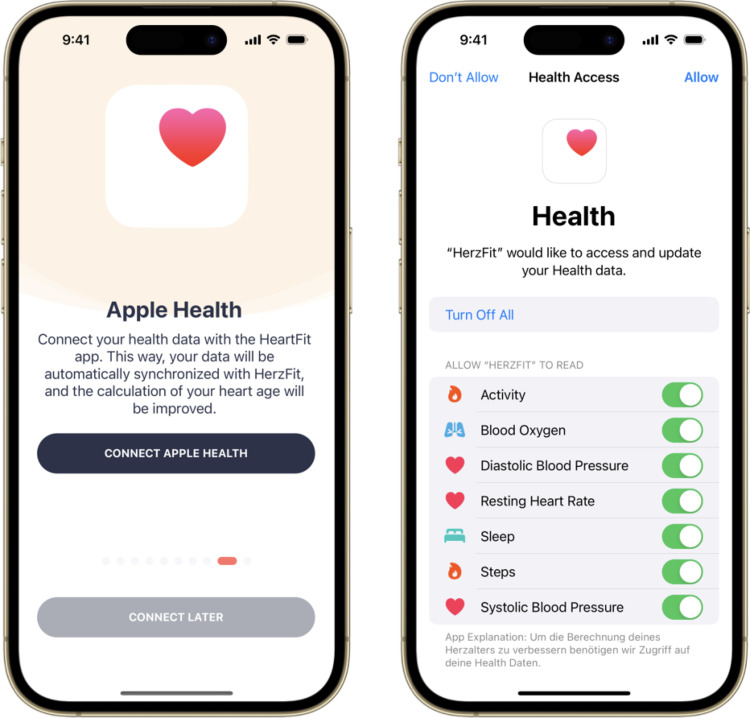
Connecting HerzFit with Apple Health/HealthKit for automatic synchronization of sensor data.
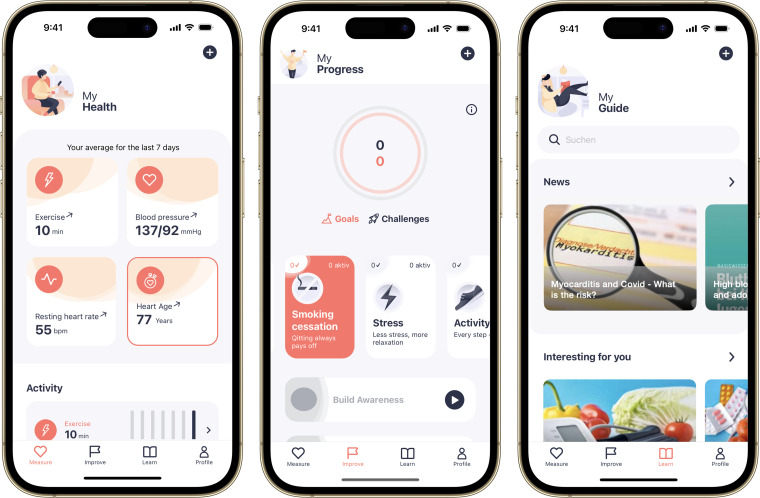
Core modules of the HerzFit app. The HerzFit app is divided into four core modules: two modules for assessing cardiovascular risk and monitoring health parameters (“Measure”), one module for lifestyle modification (“Improve”), and one module for information about cardiovascular health and disease (“Learn”). The profile area allows users to manage their profile settings and upload documents.
The “Measure” module allows individuals to record and monitor activity (exercise minutes, steps, active energy burned), body weight, vital signs (resting heart rate, blood pressure, oxygen saturation), and laboratory parameters (glycated hemoglobin, creatinine, low-density-lipoprotein-cholesterol). If parameters exceed certain ranges (e.g. systolic blood pressure>
180/110 mmHg), a warning appears. For exercise minutes, blood pressure and resting heart rate, the trend over the last seven days is additionally highlighted. Table 1 summarizes the parameters monitored in the HerzFit app.
Table 1.
Parameters monitored in the HerzFit app.
Parameter | Description | Warning |
---|---|---|
Exercise minutes (min)* | Amount of time the user spent exercising** | |
Active energy/calories burned [kcal]* | Amount of active energy the user has burned** | |
Steps* | Number of steps | |
Oxygen saturation* (%) | Oxygen saturation | <90% |
Blood pressure* (mmHg) | Systolic and diastolic blood pressure | <80/50 mmHg or >180/110 mmHg |
Resting heart rate* (bpm) | Heart rate at rest (beats per minute) | <40 bpm or >100 bpm |
Sleep* (h) | Total hours of sleep | |
BMI (kg/m2) | Body mass index | |
LDL cholesterol (mg/dl) | Low-density-lipoprotein-cholesterol | >150 mg/dl |
HbA1c (%) | Glycated hemoglobin | >7.5% |
Creatinine (mg/dl) | Parameter for kidney function | >1.5 mg/dl |
API: application programming interface.
Parameters that can be monitored in the HerzFit app. Parameters can be entered into the app either manually or automatically (*) via the operating system-specific health data storage (e.g. HealthKit on iOS). If users enter parameters that exceed certain ranges, a warning will be displayed.
For CVD risk assessment, the “Measure” module incorporates a previously described guideline-based CVD risk calculator.
31
Briefly, this calculator allows individuals to self-estimate their risk of incident or recurrent CVD based on the latest European Guidelines on CVD prevention.
1
In primary prevention, ESC SCORE2 models and a recalibrated version of the Framingham 10-year CVD score are used to estimate an individual heart age.32–35 In secondary prevention, the 10-year risk for recurrent events is estimated using the SMART risk score.
36
A comparison of the expected to mean (derived from data from the population-based Bavarian Cooperative Health Research in the Region Augsburg (KORA) cohorts F4 and FF4
37
) and optimal risk levels complete the self-assessment. Heart age and risk automatically adapt whenever risk factors change (e.g. when new cholesterol levels are entered into the app). Based on the self-assessment, individualized recommendations on lifestyle modifications and risk factor control are provided. Diabetics are advised to monitor their blood sugar, hypertensives (> 140/90 mmHg) to optimally adjust their blood pressure, and smokers to quit smoking. For diabetics, the HerzFit app additionally highlights the potential benefit of a cardiological check-up. If low-density-lipoprotein cholesterol exceeds 115 mg/dl in people without CVD or 55 mg/dl in people with established CVD, users are encouraged to better control their cholesterol levels. If users are overweight (BMI>
25 kg/m2) they are encouraged to reach their ideal body weight. Finally, every user who reports being inactive, suffering from negative stress, and not paying attention to a healthy diet will be encouraged to exercise more, avoid stress, and focus on a healthy diet.
The “Improve” module promotes lifestyle modifications regarding physical activity, healthy diet, stress reduction, and—if users are smokers—smoking cessation. Multimedia content and interactive challenges shall motivate users to optimize their lifestyles. In total, 68 tasks await the users. While some tasks simply inform the user about a healthy lifestyle, others encourage them to develop new habits over a period of several weeks. To this end, different behavior change techniques were used to both increase motivation (e.g. goal setting or reminders) and improve self-regulation (e.g. behavioral practice or restructuring the physical environment). 38 The content of the tasks was created on the basis of national (e.g. content from the German Nutrition Society and the German Center for Cancer Research) and international recommendations on healthy lifestyle.39,40
The “Learn” module offers multimedia content about cardiovascular health and diseases based on national and international recommendations and guidelines on healthy lifestyle and CVD prevention.1,7,8 The content, including articles, podcasts, and videos, was created by medical experts affiliated to the German Heart Foundation and the German Hypertension League and prepared in a way that is understandable for laypersons. Users receive information and practical tips on a healthy lifestyle, modifiable risk factors such as HTN and hypercholesterolemia, and CVD. Users are also provided with an individualized content category based on the risk assessment. Finally, a news section offers current information (e.g. dealing with temperature changes as a heart patient) and presents the latest research findings and their implications for patients.
System design and technical details
The initial system design concept for the HerzFit app was previously described. 41 Figure 2 displays HerzFit´s final system architecture. The HerzFit app receives lifestyle and health data that is either manually collected and entered by the user or automatically collected by wearables and transmitted to the app. To enable connectivity to a wide range of wearables, HerzFit communicates with the operating system-specific health data storage (e.g. HealthKit on iOS). Via this connection, HerzFit can automatically read out newly acquired sensor data at regular intervals. Hereby, we achieve a provider-independent import of sensor data. On HerzFit´s side, this creates independence from wearable manufacturers, increases robustness to application programming interface (API) changes, and enables rapid integration of new wearables. In order to guarantee its users maximum data privacy, HerzFit stores all of the user data locally on the smartphone. The content management system (CMS) is responsible for the administration and delivery of HerzFit´s content on health information and recommendations. Any update in the CMS is immediately visible in HerzFit. The CMS uses the Drupal Content Management System Framework and is written in PHP. HerzFit's content is managed by experts of the German Heart Center Munich and the German Heart Foundation and receives regular updates. This way, the content can be adapted to current guidelines at any time (Figure 4).
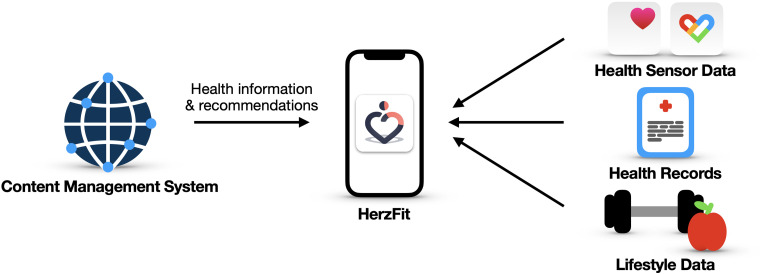
Top-level design of the HerzFit system. The HerzFit app gathers health and lifestyle data and receives content and information from the content management system.
Beta testing and usability study
From the 50 test users included in our prototype evaluation, 26 (52%) were females and 24 (48%) were males. Most of our test users were in the age range of 50 to 69 years (32% 50–59 years; 40% 60–69 years) and 84% had established CVD. When asked about the previous use of wearables, 92% reported experience with devices such as step counters or smartwatches. The usability was rated with a mean SUS score of 68.1 (Median: 70.0�
18.8), indicating the usability of the HerzFit prototype could be considered as slightly above average. In the GER-MAUQ rating, the HerzFit prototype achieved a mean score of 4.5 (Median: 4.6
�
1.4), also indicating an above-average usability. An analysis of the three subscales of the GER-MAUQ (Ease of Use, Interface and Satisfaction, and Usefulness) exposed an above-average performance in Ease of Use (Mean: 5.0, Median: 5.2
�
1.5) and Interface and Satisfaction (Mean: 4.5, Median: 4.9
�
1.7), and an average rating of Usefulness (Mean: 4.0, Median: 4.0
�
1.6) (Figure 5).
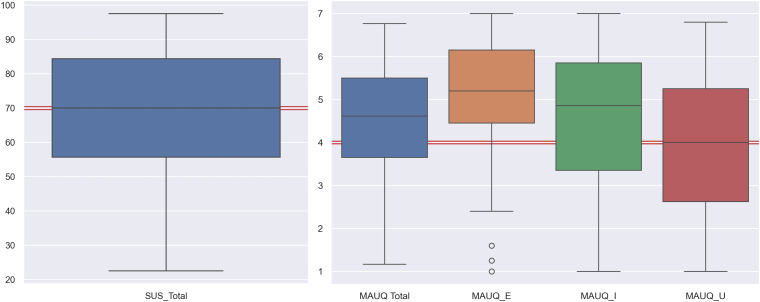
Herzfit usability testing. Boxplots of the results of the HerzFit usability testing, representing the two used questionnaires System Usability Scale (SUS) and German mHealth App Usability Questionnaire (GER-MAUQ) including its three subscales Ease of Use (GER-MAUQ_Q), Interface and Satisfaction (GER-MAUQ_I), and Usefulness (GER-MAUQ_U). With an average SUS score of 68.1 (median 70.0�
18.8) and an average GER-MAUQ score of 4.5 (median 4.6
�
1.4) HerzFit�s usability was rated as above average. In the subscale analysis, HerzFit showed an above-average performance in Ease of Use (GER-MAUQ_E) and Interface and Satisfaction (GER-MAUQ_I), and an average performance in Usefulness (GER-MAUQ_U). The average ratings for both scales are represented by the red lines.
The analysis of the free text feedback is summarized in Table 2. Participants positively noted the clarity of presentation in the HerzFit app, the variety of functionality offered, as well as the information presented in the app. Negative feedback mainly concerned problems in the automatic synchronization of wearable data or missing possibilities to connect specific wearables such as blood pressure devices. Moreover, some users considered the UI as complex and faced problems when manually entering their data. When explicitly asked for problems they experienced during the beta testing, the main feedback again concerned wearable synchronization, missing functionality for editing/deleting data, and the complexity of the date picker on Android devices.
Table 2.
Free text feedback from the usability evaluation.
Feedback item | Occurrence |
Clarity of presentation | 19× |
Functional versatility | 13× |
Variety of information in the guidebook | 11× |
Easy operation | 8× |
Calculation of the heart age/CVD risk | 6× |
Design | 4× |
(A) Positive feedback | |
Feedback item | Occurrence |
Missing interfaces or faulty synchronization of wearables leading to manual data entries | 28× |
Complex user interface | 11× |
Problems with data entry | 7× |
Visualizations of measurement values | 6× |
Missing functionality regarding drug management | 4× |
(B) Negative feedback | |
Feedback item | Occurrence |
Missing interfaces or faulty synchronization of wearables leading to manual data entries | 16× |
Correction or deletion of data missing | 4× |
Complex date selection (on Android devices) | 3× |
Problems in document upload | 3× |
Tasks in “Improve” | 3× |
(C) Problems with HerzFit |
CVD: cardiovascular disease.
Discussion
The present study illustrates the development and evaluation process of an mHealth app for CVD prevention. The main findings were as follows: user-centered design thinking led to the definition of four personas. Based on their needs, HerzFit´s functional and technical requirements as well as the evidence-based content were developed. Beta-testing and usability evaluation of the HerzFit prototype showed predominantly above-average results and enabled further technical and functional improvements to the app.
A special aspect of this study is the user-centered design approach. While design thinking is routinely used in app design outside healthcare, this approach is still underutilized in the mHealth sector.18,42 Pursuing this approach and referring to the promising results of other user-centered app developments, HerzFit´s functionality and structure were created based on the potential needs of four personas.43,44 These personas and their potential requirements were defined by our interdisciplinary team following interviews with potential app users. As HerzFit´s focus lies on supporting patients in CVD prevention, two out of the four personas represent the main target group. The other two personas (a relative of a heart patient and a physician) were selected as they represent individuals who could either use HerzFit for information or promote its usage to the main target group.
HerzFit enables its users to monitor and improve multiple cardiovascular risk factors and lifestyle habits. In contrast, many other mHealth apps available target a single risk factor.6,10,13,45,46 Positive effects on lifestyle and risk factor control were demonstrated for both types of apps.9,47,48 Yet, personalization of content seems to be particularly important for the success of apps targeting multiple factors.9,48 Therefore, HerzFit enables its users to self-assess their cardiovascular risk and receive personalized recommendations on risk factor control and lifestyle modifications. In addition, relevant content is provided in an individualized category of HerzFit´s informative module.
Referring to recent reports criticizing the lack of evidence-based content in mHealth apps, HerzFit´s content was created on the basis of national and international recommendations on healthy lifestyles and CVD prevention.1,14,31,45 The great variety of high-quality information and the guideline-based CVD risk calculator were particularly appreciated by our test users.
In our study, usability was evaluated as part of the beta testing. Test results showed mostly above-average usability of the HerzFit prototype. Users particularly appreciated the clarity of presentation as well as the functional and informational versatility. Negative feedback mainly concerned synchronization with other devices and manual data entry. At this point, it should be noted that HerzFit could only communicate with Apple Health/Health Connect at the time of the usability testing, but not yet with Google Fit/Google Health Connect. Therefore, we expect that feedback on data synchronization would be different by now. Many of the other issues we noticed through the free text feedback, such as problems with wearable synchronization, date picking on Android devices, deleting data entries and uploading documents, could also be fixed by the time HerzFit was released in April 2022 (Version 1.0.0).
Overall, the present study illustrates the user-centered development and preliminary usability evaluation of HerzFit, a multi-layered mHealth app for CVD prevention. Even though HerzFit stands out through its user-centered development approach and its comprehensive inclusion of multiple cardiovascular risk factors, several other mobile applications with similar approaches exist. As HerzFit has been specifically developed for the German-speaking market, its main competitors are to be found in Germany, Austria, and Switzerland. Of particular interest are the so-called Digitale Gesundheitsanwendungen (DiGAs, digital health applications) apps. From 2021, it is possible to prescribe these medical apps to patients in Germany. Currently, three mHealth apps targeting CVD or their risk factors are available and have already shown positive effects in randomized controlled trials 49 : actensio (HTN), 50 ProHerz (heart failure), 51 and Vantis (coronary artery disease). 52 However, these apps are not available for free and prescription requires an established diagnosis. HerzFit on the other hand, is available for free and supports both individuals with and without CVD in optimizing their risk profile.
Strengths and limitations
Our study has several strengths. We applied a user-centered design approach by defining personas and identifying their potential needs. An iterative development process was followed by a structured beta-testing and preliminary usability evaluation of the HerzFit prototype. The usability evaluation revealed a predominantly above-average performance of the prototype and enabled further improvements to HerzFit�s functionality. The app content was created on the basis of national and international guidelines and recommendations on healthy lifestyle and CVD prevention involving representatives from the largest German-speaking layperson organization. HerzFit is available free of charge in both iOS and Android app stores in Germany, Austria and Switzerland. With more than 125,000 downloads within the first 22 months, the public showed great interest in our prevention tool.
The following limitations have to be mentioned. First, the definition of personas did not follow a structured cluster analysis as reported in other studies. 42 Instead, our definition of personas was based on interviews with potential app users and subsequent discussions within our interdisciplinary team. Second, the majority of our test users reported previous CVD. Thus, the needs and interests of individuals in primary prevention might be underrepresented in HerzFit. Third, the usability testing was performed with a prototype of our final version of HerzFit. Consequently, the usability evaluation results might no longer reflect HerzFit�s current performance. Fourth, there may have been selection bias in HerzFit�s usability evaluation. Study participants wanted to use the app and might have given biased feedback. Finally, there has been no statistical evaluation of HerzFit, its user structure, and its impact on risk factor control and lifestyle behavior so far. Thus, it remains unclear whether HerzFit reaches individuals at risk and whether it helps them to better manage their health and lower the risk of incidents or recurrent CVD. However, with the technical infrastructure for anonymized data donation already being prepared, HerzFit may soon provide answers here. 41
Conclusions
The present study illustrates the user-centered development of a guideline-based mHealth app for CVD prevention. Beta-testing and a preliminary usability study were used to improve HerzFit until its official release in April 2022.
Supplemental Material
Supplemental material, sj-docx-1-dhj-10.1177_20552076241249269 for User-centered development of an mHealth app for cardiovascular prevention by Lara Marie Reimer, Leon Nissen, Moritz von Scheidt, Benedikt Perl, Jens Wiehler, Sinann Al Najem, Florian P. Limbourg, Theodora Tacke, Angelina M�ller, Stephan Jonas, Heribert Schunkert and Fabian Starnecker in DIGITAL HEALTH
Acknowledgements
The authors would like to thank the Techniker Krankenkasse and the German Hypertension League for their support in HerzFit�s development.
Footnotes
Contributorship: LR and FS wrote the first draft of the manuscript; LR, LN, MVS, BP, JW, SAN, FL, HS, SJ, and FS contributed to the conceptualization and implementation of the HerzFit app; TT and AM conceptualized the usability questionnaires; LR conducted the usability study and evaluated the results. All authors reviewed and edited the manuscript and approved the final version of the manuscript.
The authors have nothing to disclose.
Ethical approval: The usability testing of the HerzFit app was approved by the local ethics committee of the Technical University of Munich (REC number 636/21 S-SR). Each participant provided signed, written informed consent before being allowed to participate in the usability testing process.
Funding: The authors reported support from the Bavarian State Ministry of Health and Care through the research project DigiMed Bayern. MVS reported support from the Clinician Scientist Excellence Programme of the German Center for Cardiovascular Research (DZHK), the German Society of Cardiology (DGK), and the German Heart Foundation.
ORCID iDs: Benedikt Perl https://orcid.org/0009-0001-6606-3384
Jens Wiehler https://orcid.org/0009-0007-0121-240X
Theodora Tacke https://orcid.org/0009-0007-7031-8427
Angelina Müller https://orcid.org/0000-0003-0162-7000
Stephan Jonas https://orcid.org/0000-0002-3687-6165
Fabian Starnecker https://orcid.org/0000-0001-9850-4239
Supplemental material: Supplemental material for this article is available online.
References
Articles from Digital Health are provided here courtesy of SAGE Publications
Similar Articles
To arrive at the top five similar articles we use a word-weighted algorithm to compare words from the Title and Abstract of each citation.
The German version of the mHealth App Usability Questionnaire (GER-MAUQ): Translation and validation study in patients with cardiovascular disease.
Digit Health, 10:20552076231225168, 31 Jan 2024
Cited by: 1 article | PMID: 38303970 | PMCID: PMC10832428
The mHealth App Usability Questionnaire (MAUQ): Development and Validation Study.
JMIR Mhealth Uhealth, 7(4):e11500, 11 Apr 2019
Cited by: 208 articles | PMID: 30973342 | PMCID: PMC6482399
German Version of the mHealth App Usability Questionnaire in a Cohort of Patients With Cancer: Translation and Validation Study.
JMIR Hum Factors, 10:e51090, 01 Nov 2023
Cited by: 3 articles | PMID: 37910144 | PMCID: PMC10652191
Tools for Evaluating the Content, Efficacy, and Usability of Mobile Health Apps According to the Consensus-Based Standards for the Selection of Health Measurement Instruments: Systematic Review.
JMIR Mhealth Uhealth, 9(12):e15433, 01 Dec 2021
Cited by: 23 articles | PMID: 34855618 | PMCID: PMC8686474
Review Free full text in Europe PMC